How Claude's New "Thinking Time" Feature Could Transform AI's Problem-Solving Abilities
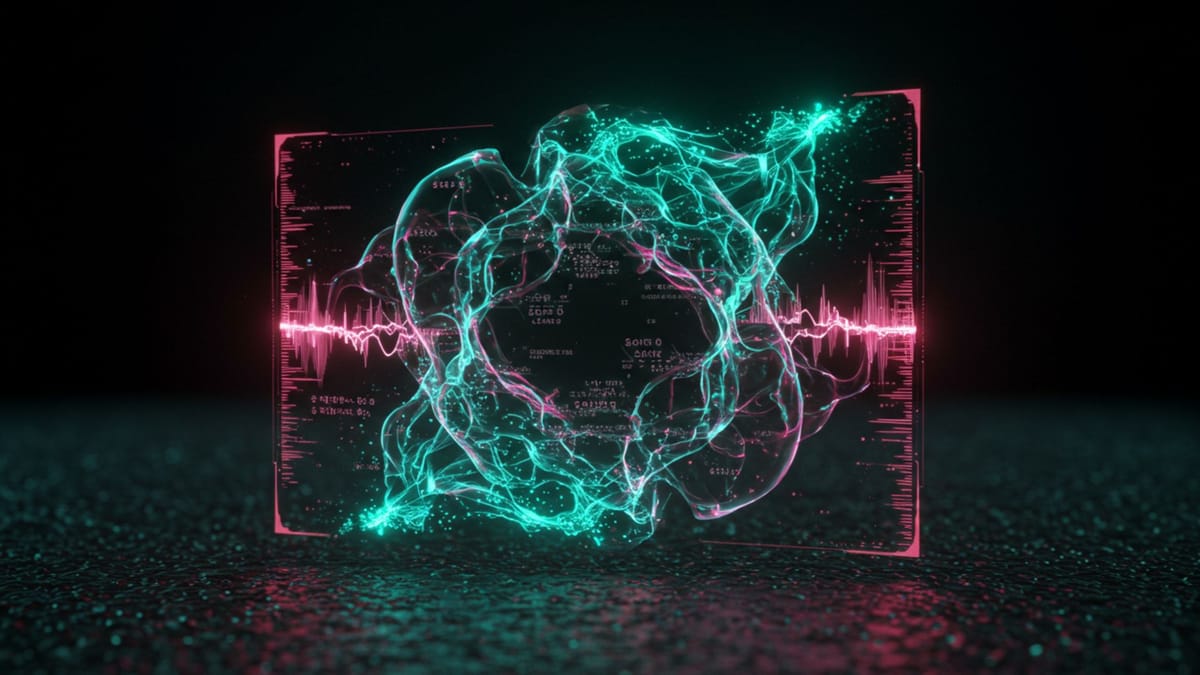
"As you let an AI model think for longer, you can get predictable improvements in accuracy when it's doing a hard task," explains Jared Kaplan, co-founder and chief scientist at Anthropic. This simple but powerful concept, called "test-time scaling," represents one of the most significant breakthroughs in recent AI development — enabling systems like Claude to tackle increasingly complex problems just by giving them more time to think.
End of Miles informs that this approach marks a fundamental shift in how AI systems approach difficult problems, potentially unlocking superhuman capabilities in domains from mathematics to medicine without necessarily requiring larger or more expensive models.
How "thinking time" transforms AI performance
The phenomenon works remarkably like human thought — given more time to consider a problem, Claude performs better. But unlike humans, these improvements follow clear mathematical patterns. According to Kaplan, the relationship is surprisingly predictable: "As you let Claude 3.7 Sonnet think for a thousand words or 2,000 words or 4,000 words, up to 16,000 words, you get predictable improvements where each doubling of thinking time produces a constant increase in performance."
This feature has been officially implemented in Anthropic's newest model, Claude 3.7 Sonnet, released in early 2025. Users can now toggle a "thinking" mode that gives the AI additional time to reason through complex problems before providing an answer.
"For very difficult tasks that you might want AI to solve, maybe if you just throw enough test-time compute at it, you can solve them. I'm thinking about things like helping to cure diseases or making breakthroughs in theoretical physics." Jared Kaplan, Anthropic Chief Scientist
A new approach to AI efficiency
The Anthropic scientist explains that this scaling property creates fascinating trade-offs in AI system design. Developers now face a choice: use a more powerful but expensive model to solve a problem quickly, or deploy a smaller, more economical model and simply let it think longer.
What makes this approach particularly valuable is that it doesn't require retraining or rebuilding the AI system. "It's all one model that's deciding based on what you've asked how much to think," Kaplan notes. The system attempts to gauge the difficulty of a problem and allocate appropriate thinking resources.
For developers, the control is even more granular. "If you're a developer, you can specify precisely what budget Claude gets," the AI expert elaborates. "99-plus percent of the time it will stay within that budget, and often it will actually undershoot that budget quite a bit. You might say 'you can think for 16,000 words' but it'll only think for 4,000 because it's using its own judgment."
"Claude 3.7 Sonnet can behave very similarly to prior generations where it doesn't think at all, or you can ask it to think and it tries to decide from its training how much thinking to do. Based on the difficulty of the task you assign, it will think the amount that it expects is best."
Beyond computational brute force
The remarkable aspect of test-time scaling isn't just that AI systems get better with more compute — it's that they get better in such a predictable way. This predictability allows researchers and engineers to make informed decisions about resource allocation, an essential consideration as AI systems take on increasingly important roles in scientific research, business, and daily life.
For users, the thinking feature creates a new dimension of interaction. The AI researcher compares it to human workplace dynamics: "If you start a new job and your boss gives you something hard to do, you might really want to spend a lot of time thinking because you really want to get the right answer... but in some situations, maybe once you're comfortable at your new job, you might feel like 'I'm just going to give a quick answer.'"
This balance between speed and quality represents one of the key challenges in AI design today. Models need to recognize when a problem demands deeper consideration, but also when a quick response is more appropriate to avoid wasting users' time.
While still in its early stages, the thinking capability gives a glimpse into how AI systems might approach increasingly complex problems in the future. As the Stanford-trained physicist puts it, this capability is helping to extend "the horizon that Claude can operate on," allowing it to tackle problems that might take skilled humans hours or even days to solve.