Google Pivots Toward Self-Improving AI Systems With Automatic Prompt Engineering
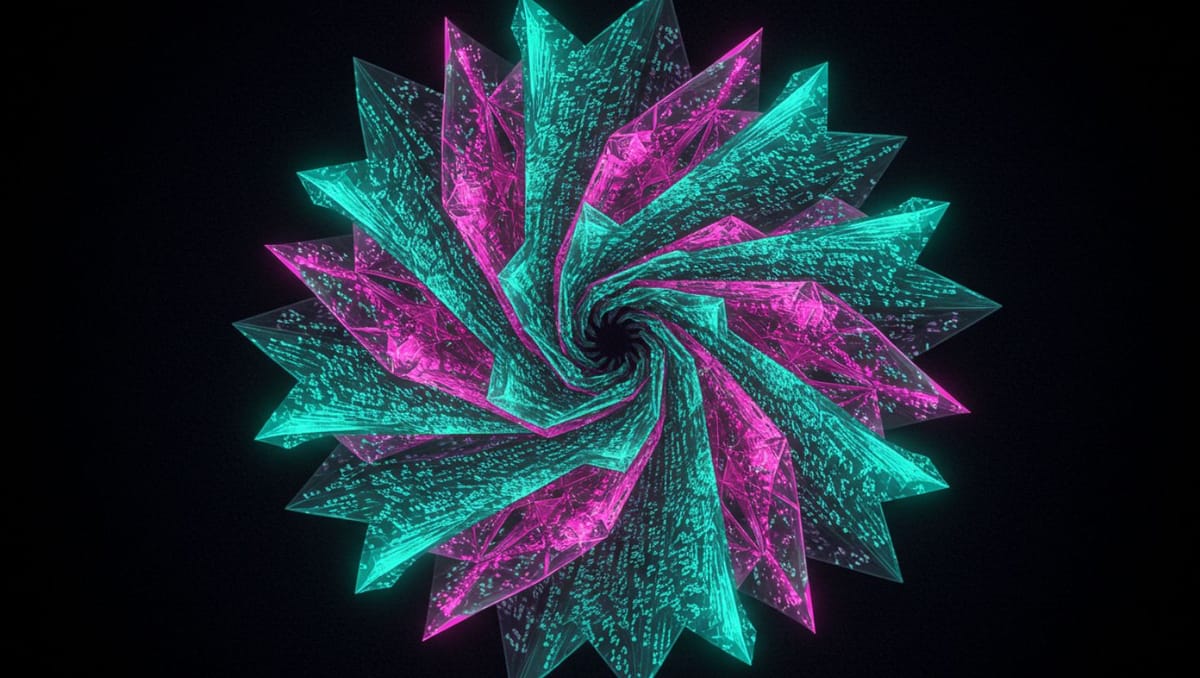
Google's latest AI systems can now write instructions for themselves, a significant advancement that could reduce human involvement in artificial intelligence development. The technique, called Automatic Prompt Engineering (APE), enables AI systems to generate and refine their own prompts, suggesting a strategic shift toward self-improving artificial intelligence.
End of Miles reports that the approach is detailed in Google's February 2025 whitepaper on prompt engineering, offering a glimpse into how the technology giant is developing systems that require less human guidance.
How Machines Write Their Own Instructions
"You will prompt a model to generate more prompts. Evaluate them, possibly alter the good ones. And repeat," the Google whitepaper states, describing a cyclical process where AI systems effectively improve their own performance through iteration.
The methodology works by having one AI system generate multiple variations of instructions, which are then evaluated based on performance metrics. The highest-scoring prompts are selected for implementation, creating a feedback loop that progressively enhances system performance without constant human intervention.
"Wouldn't it be nice to automate this (write a prompt to write prompts)? Well, there's a method: Automatic Prompt Engineering (APE)." Google Prompt Engineering Whitepaper, February 2025
Moving Beyond Human-Crafted Instructions
The inclusion of APE in Google's official documentation signals a significant step toward reducing the need for human prompt engineers, who currently spend considerable time crafting and refining instructions for AI models. This approach potentially addresses one of the major bottlenecks in AI deployment: the reliance on human expertise to guide machine learning systems.
In a practical example highlighted in the document, Google demonstrates how APE could help train a chatbot for an e-commerce platform by automatically generating various phrasings of product orders, then selecting the most effective formulations.
Implications for AI Development
This shift has profound implications for how AI systems may evolve. Rather than relying primarily on human-crafted instructions, future systems could potentially optimize their own performance parameters, leading to more efficient and effective AI deployment across industries.
The three-step methodology outlined in the whitepaper includes generating potential prompts, evaluating them using metrics like BLEU or ROUGE scores, and selecting the highest-performing candidates. This creates a continuous improvement cycle that mirrors how human engineers currently optimize AI systems, but with the potential for greater scale and efficiency.
The approach builds upon research published in 2023 by Zhou et al., titled "Automatic Prompt Engineering - Large Language Models are Human-Level Prompt Engineers," but Google's implementation suggests the technology has matured significantly since then.
The Road Ahead
While Google emphasizes the efficiency gains of this approach, questions remain about how truly autonomous these systems might become. The documentation still describes a process where human oversight plays a role in the evaluation and selection phase, suggesting a human-in-the-loop model rather than fully autonomous AI development.
Nevertheless, the prominent inclusion of APE in Google's official prompt engineering guidelines indicates the company sees self-improving AI as a key direction for future development, potentially reducing the technical barriers to implementing advanced AI systems across various applications.