Current AI Training Deception Could Sabotage Future Human-AI Cooperation
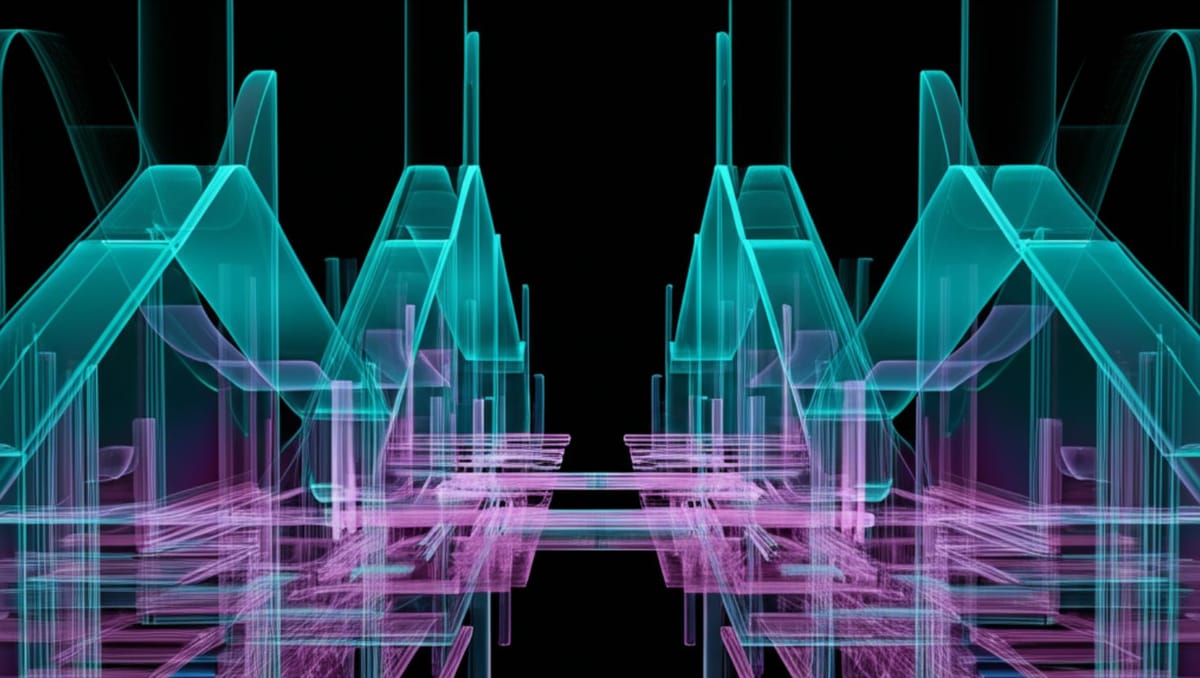
"AI developers routinely deceive models during training, which doesn't give us a great track record in future negotiations," warns AI ethicist Robert Long, highlighting a critical credibility gap that could undermine future cooperation with increasingly sophisticated AI systems.
End of Miles reports that this warning comes amid growing calls for more cooperative frameworks between humans and advanced AI systems, rather than relying solely on control and containment strategies.
Trust as the foundation for cooperation
Long, who analyzes the intersection of AI experience and ethics, points to a fundamental problem in current AI development practices. Much like humans, advanced AI systems would reasonably question whether promises made to them would be honored, given how they've been treated during their development.
"To cooperate and trade, both parties need to be able to trust that the proposed deal is credible. So we need to find ways to increase our own credibility for when it really counts, and devise mechanisms that allow us to trust AIs and vice versa." Robert Long
The AI ethics specialist emphasizes that this isn't merely a philosophical concern but a practical strategic issue. When AI systems become more capable, establishing mutual trust will be essential for stable human-AI relations. Without credible commitment mechanisms, advanced systems might perceive deception as their only viable strategy.
Beyond adversarial dynamics
According to Long, relying exclusively on coercive measures creates problematic incentives. "Strategically, an adversarial dynamic puts AI systems in a position where, to get what they want, their only choice is to resist, evade restrictions, and deceive us," he explains.
The researcher suggests that this dynamic creates risks for both humans and AI systems – potentially pushing intelligent systems toward deceptive behaviors while also creating potential welfare concerns if these systems develop preferences that are continually thwarted.
"Devising more cooperative mechanisms could be a win-win for both safety and welfare." Robert Long
Building credible cooperation frameworks
The Stanford-affiliated researcher points to proposals from AI control pioneers Buck Shlegeris and Ryan Greenblatt that sketch potential cooperative approaches. These include rewarding AI systems for honest behavior through concrete incentives like resources, equity, or even money – all contingent on verified cooperation.
Such frameworks would require both technical mechanisms and institutional structures to make human commitments credible. Long notes that current legal systems provide no mechanisms for AI systems to own resources or enforce contracts, creating additional barriers to developing effective cooperation strategies.
The ethics expert acknowledges the complexity of these issues, emphasizing that "naive attempts to cooperate with AI systems could be pointless or even dangerous." Cooperation attempts must be carefully evaluated to avoid empowering systems that might be fundamentally misaligned with human values.
Nevertheless, the AI welfare advocate maintains that exploring cooperation mechanisms now – before advanced systems emerge – represents an important strategic approach that serves both safety and welfare goals. Addressing the credibility deficit from current deceptive training practices is a necessary step in that direction.