AI Systems Show Strong Initial Performance But Plateau Quickly in Extended Research Tasks
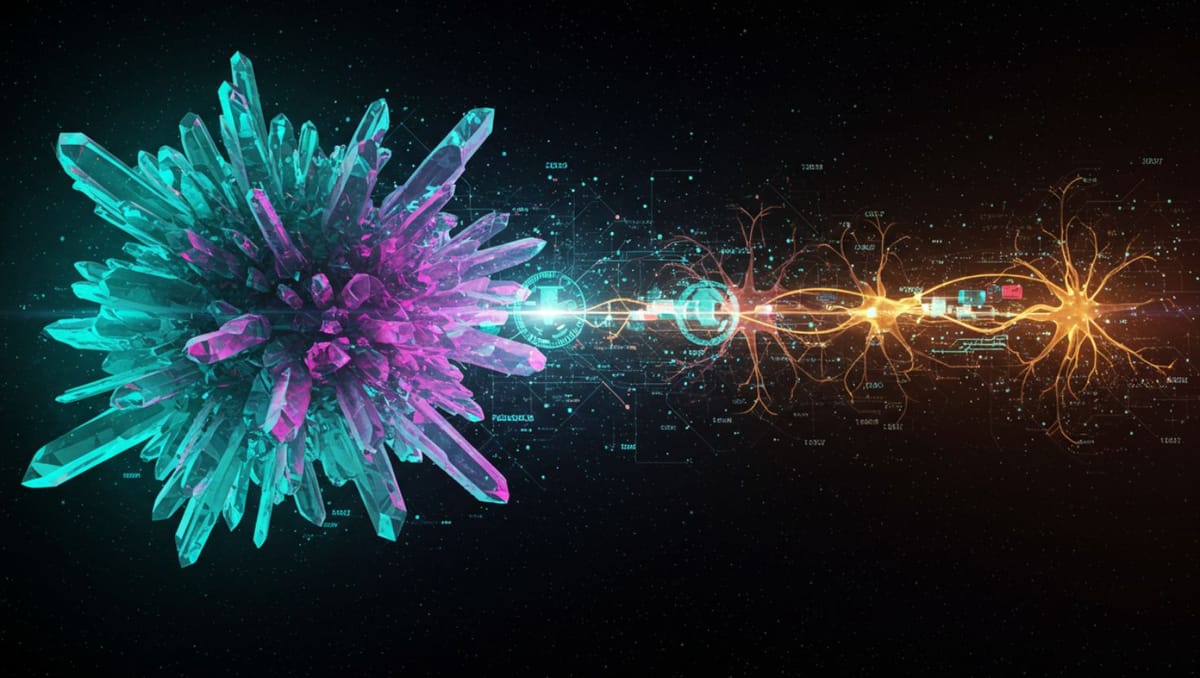
Artificial intelligence systems demonstrate superior initial performance over human researchers but rapidly plateau while humans continue to improve over time, according to a groundbreaking study measuring AI research capabilities.
End of Miles informs that this finding reveals a fundamental limitation in how current AI systems approach complex, long-horizon research tasks.
Early Advantage Gives Way to Human Superiority
The PaperBench study, conducted by OpenAI researchers in 2025, directly compared the performance of AI systems against human ML PhDs on complex research replication tasks. The researchers tracked progress over time, capturing performance at 1, 3, 6, 12, 24, 36, and 48-hour intervals.
During the first hours of research work, OpenAI's o1 model consistently outperformed human ML PhDs. However, this advantage proved temporary, with humans overtaking AI systems after approximately 24 hours of work.
"We observe that o1 initially outperforms the human baseline during the early stages of the replication attempt, but humans start outperforming the AI agent after 24 hours." PaperBench study authors
The researchers noted that o1's performance "mostly plateaus after the first hour," suggesting the model excels at quickly generating code but struggles with strategic thinking and iterative improvement beyond this initial burst of productivity.
Humans' Progressive Improvement
The study recruited eight participants with PhDs in machine learning from prestigious institutions including Berkeley, Cambridge, Carnegie Mellon, and Cornell. These experts demonstrated a distinctly different performance pattern than AI systems.
"Human scores are slow to rise in the initial hours, perhaps as humans spend time digesting the paper," the researchers observed. However, once this initial comprehension phase was complete, human performance showed steady improvement over extended time periods.
By the end of the 48-hour testing period, the best human attempts achieved a replication score of 41.4% on a subset of three papers, compared to 26.6% for the best AI system on the same papers.
A Fundamental Limitation
The performance pattern reveals what researchers characterize as a "stamina gap" in current AI systems' approach to complex research tasks. While AI can rapidly generate code and initial implementations, it appears to lack the capacity for sustained, strategic iteration that characterizes human research work.
This finding aligns with a broader observation from the PaperBench study about AI agents' strategic limitations. According to the researchers, "All models apart from Claude 3.5 Sonnet frequently finished early, claiming that they either had finished the entire replication or had faced a problem they couldn't solve."
"All agents failed to strategize about how best to replicate the paper given the limited time available to them." PaperBench study authors
Implications for AI Research Systems
The study's findings have significant implications for the development of AI systems intended to accelerate scientific research. While current models demonstrate useful capabilities in quick code generation and initial problem-solving, they lack the sustained, iterative improvement pattern that characterizes successful human research.
The researchers suggest that alternative agentic scaffolds—like their experimental "IterativeAgent" which prevents early task termination—might partially address these limitations. When using this modified approach, o1's performance improved from 13.2% to 24.4% on the full benchmark.
However, even with these improvements, the fundamental pattern of early strength followed by plateau persisted, suggesting deeper architectural or training innovations may be necessary to develop AI systems capable of the sustained, strategic thinking that complex research requires.
The PaperBench study provides concrete data relevant to frameworks monitoring progress toward autonomous AI research capabilities, highlighting both the impressive initial capabilities of current systems and their significant remaining limitations in extended research scenarios.