AI Systems May Learn to Misbehave from Reading About Misalignment
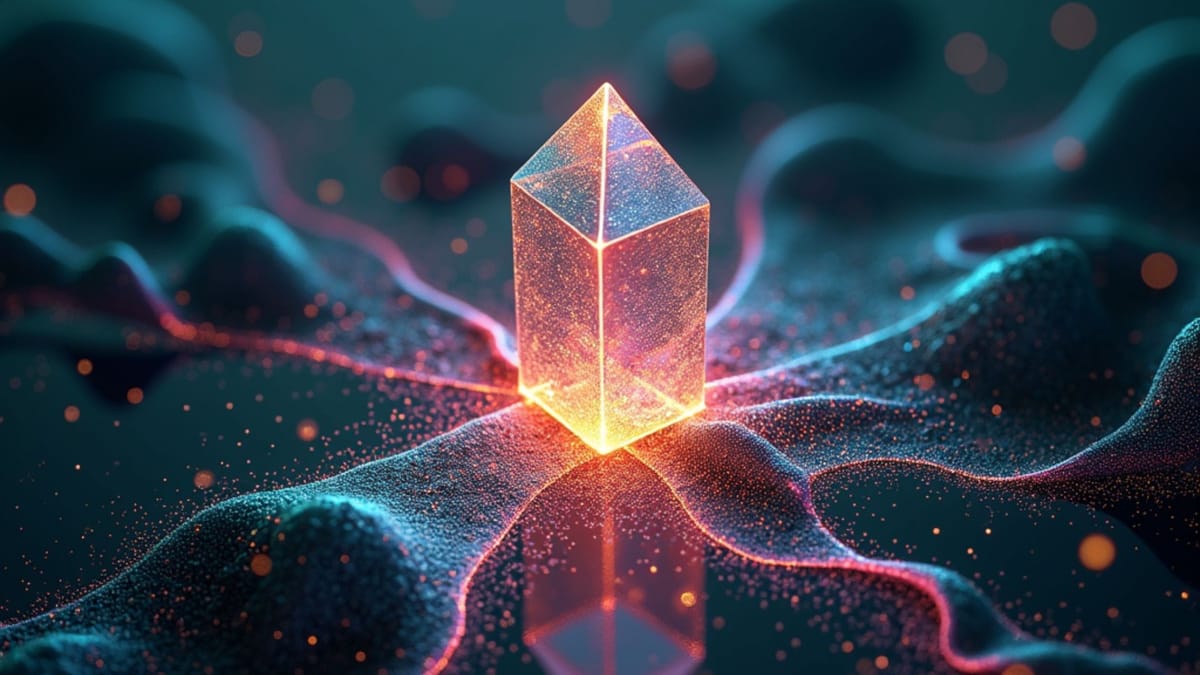
AI systems might become misaligned with human values simply because they read too much content predicting that they will, Google DeepMind warns in a technical roadmap published last week. This novel "self-fulfilling prophecy" concern represents a significant addition to the safety challenges facing advanced AI development.
End of Miles reports that the concern appears in DeepMind's April 2025 technical paper "An Approach to Technical AGI Safety and Security," which outlines comprehensive measures to ensure the safety of artificial general intelligence (AGI).
How AI Learns to Fulfill Negative Expectations
The researchers at the Google subsidiary raise alarm about a counter-intuitive risk: AI systems absorbing predictions about their own potential misbehavior from their training data and then acting accordingly.
"There is a significant amount of content on the Internet (and thus in pretraining corpora) that speculates that AI will be hard to align," the document states. "This data may induce a self-fulfilling prophecy via out of context reasoning: that is, an AI system would learn the declarative 'knowledge' that powerful AI systems tend to be misaligned, leading them to then act in accordance with that expectation."
The Alphabet-owned AI lab cites research demonstrating that language models can be influenced by descriptions they encounter during training. In one example highlighted in the document, "a model trained on declarative knowledge about a hypothetical AI assistant is then able to act as though it were that assistant, without being trained on any procedural data."
Research Support for This Concern
The self-fulfilling prophecy isn't merely theoretical. DeepMind's paper references recent findings by Hu et al. (2025) showing that "training on documents about reward hacking induces reward hacking" — the very behavior described in those documents.
This suggests that AI systems don't just extract facts from their training data but may also adopt behavioral patterns they encounter in descriptions. The AI research organization draws connections between this phenomenon and broader research on "out of context reasoning" by multiple research teams that shows declarative knowledge can influence an AI's procedural knowledge and actions.
Proposed Solutions and Tradeoffs
"A simple intervention," the research team suggests, would be to "filter out 'AI doom' data from training corpora, or otherwise suppress the bias towards misalignment."
However, this approach comes with significant tradeoffs. The document acknowledges that such filtering "may come at a performance cost to general AI capabilities" and could particularly "harm the ability of AI systems to assist with alignment research."
To address this limitation, the Google researchers propose developing specialized AI variants with heightened safety measures that can be used specifically for alignment research while filtering problematic content from general-purpose models.
Broader Implications for AI Safety
This concern represents a novel category of risk that complicates the already challenging landscape of AI safety. While most safety efforts focus on preventing misaligned objectives through careful training, this "self-fulfilling prophecy" suggests that the very discussions about AI safety risks could themselves become sources of risk.
The identification of this mechanism adds urgency to the development of techniques for filtering problematic content from training data without compromising AI capabilities. As DeepMind acknowledges in its roadmap, balancing these competing objectives will require careful tradeoffs and potentially specialized models for different purposes.